A trial for regression model building and diagnostics for prediction purposes
Ключевые слова:
прогнозирование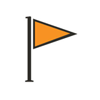
Приглашаем к сотрудничеству авторов научных статей
Публикация научных статей по экономике в журналах РИНЦ, ВАК (высокий импакт-фактор). Срок публикации - от 1 месяца.
creativeconomy.ru Москва + 7 495 648 6241
On the basis of empirical data concerning a chemical product sales a pro-cess of model construction begun. The desire of the company – seller was to improve its forecasting activity. The initial list of the variables intended to be included in the model was as following:y: the demand for the product in a certain sales period in thousands of bottles;x1: the price in EUR of the product as offered by the seller;x2: the average branch price in EUR of competitors similar products;x3: the company‘s advertising expenditure (in thousands of EUR) to promote the product;x4 = x2 – x1: the “price difference”.Information regarding demand for the product was gathered over 30 sales periods (each sales period is defined to be a four - week period). In other words the model was based on 30 observations. It is quite explainable that y – varia-ble is the dependent, a direct subject of prediction. The other variables play the role of predictors.We considered that the single independent variable x4 adequately describes the effects of x1 and x2 on y. This makes since recent researches showed that most buyers must purchase a certain amount of the product no matter what the price might be. At the same time product demand depends strongly on how the price of the product compares to competitors prices.The scatter plots of y versus x4 and x3 indicates:a) y tends to increase in a linear fashion as x4 increases;b) y tends to increase in a curvilinear fashion as x3 increases.In order to construct an appropriate prediction model based on both x3 and x4, it seems reasonable to use the following relationship:Y = bo + в1х4 + в2х3 + в3 .
The least squares point estimates of the model parameters are:вo = 17,3244; в1 = 1,3070; в2 = - 3,6956; в3 = 0, 3486. At preliminary cho-sen level of significance = 0,05 the p – values related to x4, x3 and are 0,0002, 0,0564 and 0,0293 respectively. Therefore, there is strong evidence that each of the model components x4 and is significant. Although the p – value related to x3 is slightly greater than 0,05 we retained x3 in the model because is significant.The model showed adjusted coefficient of determination = 0,8945 – a quite acceptable value. Of course, it was more important for us to achieve a small standard error of regression. It wasn’t clear that a number 0,2213 wood be sufficiently small.That‘s why further trials for model‘s improvement were made. For exam-ple, a new variable illustrating the possible interaction between x4 and x3 was involved. The positive result didn‘t delay. The standard error decreased to 0,2063. At the same time explainational ability of the model expressed by increased to 0,9209.Before to use the model for predictive aims it was necessary: a) to check for multicolinearity between independent variablesb) to conduct a complete residual analysisFortunatly, serious departures from the regression assumptions weren‘t detected. All this allowed us to recommend the model for practical needs.
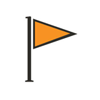
Издание научных монографий от 15 т.р.!
Издайте свою монографию в хорошем качестве всего за 15 т.р.!
В базовую стоимость входит корректура текста, ISBN, DOI, УДК, ББК, обязательные экземпляры, загрузка в РИНЦ, 10 авторских экземпляров с доставкой по России.
creativeconomy.ru Москва + 7 495 648 6241
2. Bowerman, Bruce L and Richard T.O.Connel, Linear Statistical Mo-dels: An Applied Approach, Boston, MA: PWS – KENT, 2000
3. Gorman, J.W. Selection of Variables for Fitting Equations to Data, Technometrics, 1996
A trial for regression model building and diagnostics for prediction purposes. , 2-4.